Effective Data Governance in Data Spaces
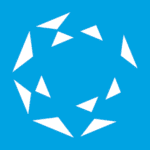
Executive Summary: This guide explores data space governance, emphasizing its role in fostering innovation, ensuring compliance, and driving sustainable growth through ethical engineering practices. Key topics include the importance of data governance, essential components, the data space concept, implementation strategies, and building a culture of data stewardship.
Data governance is the framework of policies, processes, and standards that ensure the effective and efficient use of information across an organization, enabling better decision-making and regulatory compliance. or data space. As organizations and data collaboratives increasingly rely on data to drive innovation, enhance decision-making, and fuel economic growth, the need for robust data governance frameworks becomes paramount. This article explores the world of data governance, focusing on data space concepts and offering a comprehensive guide on establishing effective data governance practices for organizations committed to solving global challenges through technology.
The Importance of Data Governance
-
Enhancing Decision-Making: Data governance ensures that data is accurate, complete, and reliable, which enhances decision-making processes. Organizations can leverage high-quality data to make informed strategic decisions, optimize operations, and drive business value.
-
Compliance and Risk Management: With the rise of regulations such as GDPR and the Data Governance Act, organizations must ensure compliance with legal standards. Data governance frameworks help mitigate risks by ensuring data privacy and protection, thereby safeguarding against potential regulatory fines and reputational damage.
-
Fostering Innovation: By establishing clear data governance policies, organizations can foster innovation through data sharing and collaboration. Data governance facilitates the creation of data collaboratives where organizations can share data securely, unlocking new insights and opportunities for innovation.
How do data governance frameworks enhance decision-making?
Data governance frameworks enhance decision-making by providing a structured approach to managing data across its lifecycle. By establishing clear policies, roles, and controls, organizations can ensure that data is accurate, consistent, and secure, enabling more confident and strategic decisions. The EU Data Governance Act (DGA) reinforces this by creating a legal foundation for trustworthy data sharing across sectors and borders—ensuring that both public and private entities handle data responsibly. These frameworks also reduce risk by aligning with compliance standards, promoting transparency, and embedding ethical principles into everyday data use—leading to decisions that are not only data-driven, but also legally sound and socially responsible.
Key Components of Data Governance
-
Establishing Governance Principles: Organizations should define core principles that guide data access and use. These principles should emphasize responsibility, accountability, transparency, and equity throughout the data lifecycle. Engaging stakeholders in participatory working groups can enhance adherence and buy-in to these principles.
-
Developing Regulatory Frameworks: To balance innovation with data rights, comprehensive regulatory frameworks are essential. These frameworks provide a foundation for data collaboratives, ensuring data protection while promoting responsible data (re)use.
-
Cultivating Data Stewardship: Data stewards play a pivotal role in data governance. They bridge the gap between data science and policy, ensuring data is used responsibly and ethically. Investing in data stewardship capabilities is crucial for sustaining data value and fostering cross-sector data collaboration.
-
Investing in Data Infrastructure: Robust data infrastructure supports data collection, storage, maintenance, and sharing. Establishing standardized data formats and secure infrastructures ensures data quality and reliability, enabling effective data governance.
-
Data Quality Management: Implement robust data quality management processes to ensure data accuracy, completeness, and consistency. This includes regular data audits, cleansing procedures, and the establishment of data quality metrics.
-
Ethical Considerations: Incorporate ethical guidelines into data governance frameworks, ensuring that data use aligns with principles of fairness, transparency, and social responsibility. This is crucial for organizations aiming to solve global challenges through technology.
What Are The Key Components of Data Governance Frameworks?
People: A strong framework begins with clearly defined roles and responsibilities. This often includes a steering committee and key figures like a Chief Data Officer to establish data policies and standards. Operational roles—such as data stewards and data architects—are responsible for implementing these policies and ensuring proper data handling throughout the organization. The IDSA Functional Requirements offer a comprehensive overview of how these roles are structured within federated data spaces, supporting decentralized but coherent governance.
Processes: Clear and well-documented processes ensure that governance policies are enforced. These include controls for data privacy, security, integrity, and lifecycle management to ensure compliance and consistency across systems and teams.
Technology: Governance is further supported by tools such as metadata management platforms, policy automation engines, and monitoring solutions. Increasingly, technologies like machine learning are being used to support anomaly detection, automate classification, and uphold governance standards at scale.
Data Space Concept
A data space is a decentralized infrastructure designed for trustworthy data sharing and exchange within data ecosystems. It is built around commonly agreed principles and standards, enabling effective and trusted data sharing among participants. According to the International Data Spaces Association (IDSA) and the European Commission, a data space is a virtual environment that provides a standardized framework for data exchange, based on common protocols and formats, as well as secure and trusted data sharing mechanisms.
Key Characteristics
Decentralized Nature: Data spaces are characterized by their decentralized architecture, where data is not stored centrally but rather at the source, ensuring data sovereignty and allowing data owners to retain control over their data.
Standardization and Interoperability: Designed to be interoperable with other data spaces, enabling seamless data exchange across different domains and industries through standardized frameworks like the IDS Reference Architecture Model (IDS RAM).
Security and Trust: Emphasizing security and trust, data spaces have mechanisms in place to ensure secure data exchange, guided by frameworks such as the IDSA Rulebook.
Technology Agnosticism: Data spaces are technology-agnostic, allowing for data portability regardless of the platform on which the data is hosted or consumed.
Role in Data Governance
Data Sovereignty: Data spaces maintain data sovereignty by ensuring that data owners retain control over their data, determining who can use it and under what conditions.
Compliance and Governance: Governance frameworks associated with data spaces help ensure compliance with regulatory environments, enhancing trust and security in data governance.
Interoperability and Integration: By facilitating seamless data exchange, data spaces enhance the efficiency and effectiveness of data governance, enabling comprehensive and informed decision-making.
Image from Data Spaces Support Centre
Implementing Data Governance: A Step-by-Step Approach
-
Define the Problem and Purpose: Begin by identifying the specific issue data governance is intended to address. Clarifying the purpose and value proposition behind data access and reuse is key to aligning stakeholders and allocating resources effectively. As emphasized in the EU Data Governance Act, “the purpose of this Regulation is to foster the availability of data for use by increasing trust in data intermediaries and by strengthening data-sharing mechanisms across the Union.” This reinforces the foundational role of trust and shared purpose in any governance initiative.
-
Map Data Demand and Supply: Conduct a pre-feasibility analysis to identify data needs and sources. Determine the minimum viable dataset required to address the problem, ensuring data availability and accessibility.
-
Design Collaborative Models: Select appropriate data collaborative models that fit the data governance goals. Consider factors such as data accessibility, collaboration dynamics, and the scope of data sharing initiatives.
-
Monitor and Evaluate: Implement a monitoring and evaluation framework to assess the impact of data governance practices. Regularly review data use, stewardship practices, and collaborative models to ensure they meet organizational goals and regulatory standards.
-
Challenges and Mitigation Strategies: Anticipate common challenges in data governance implementation, such as resistance to change, lack of executive support, and data silos. Develop strategies to address these challenges, including change management programs, securing executive sponsorship, and promoting cross-departmental collaboration.
What Are The Main Challenges in Implementing Data Governance?
Implementing data governance in organizations involves several key challenges that can significantly hinder the effective use of data as a strategic asset.
-
Understanding the Business Value: Many organizations struggle to see the value of data governance. To address this, appointing a Chief Data Officer (CDO) and involving analytics groups can help demonstrate the importance of governance in improving data quality and supporting strategic decisions.
-
Ownership Misconceptions: A common misconception is that data ownership belongs solely to IT. Effective data governance shifts this responsibility to business managers, supported by data stewards who work alongside IT to manage access and ensure quality.
-
Resource Allocation: Governance often stalls due to underinvestment or poorly allocated roles. Organizations should invest in training or hire experts like Data Stewards to provide focused attention on governance tasks.
-
Siloed Data: Disconnected systems and teams lead to siloed data, impeding integration. Tools like metadata management and semantic models can help unify data. As the IDSA Functional Requirements note, “clear assignment of responsibilities, harmonized interpretation of policy rules, and semantic alignment are essential to enable interoperability and trusted data sharing.”
-
Poor Data Quality: Low-quality data creates mistrust. Data intelligence tools can monitor and enhance data quality, fostering confidence and broader adoption.
-
Poor Data Context: Without proper context, data can be misunderstood. Feedback loops and clear documentation help users understand how data should be interpreted.
-
Lack of Data Control: Excessive control can restrict data utility, while lax controls increase risk. A strong governance framework strikes a balance—protecting sensitive information while ensuring accessibility for legitimate use.
Building a Culture of Data Governance
-
Leadership and Culture: Leadership plays a critical role in fostering a data-driven culture. Encourage leaders to advocate for data stewardship and collaboration, embedding data governance practices across the organization.
-
Engaging Stakeholders: Cultivate a social license for data (re)use by engaging with stakeholders, including the public, to build trust and transparency. Use participatory approaches like citizen assemblies to gauge public opinion and ensure data governance aligns with societal values.
Conclusion
Effective data governance is a critical business function that goes beyond compliance; it is about creating value, fostering innovation, building trust, and ultimately driving competitive advantage in today's data-driven landscape. By implementing robust data governance frameworks, organizations can navigate the complexities of the data landscape, unlocking the full potential of their data assets while safeguarding against risks. As the digital landscape continues to evolve, staying ahead with strategic data governance practices will be key to maintaining a competitive edge and driving sustainable growth.
This comprehensive guide draws from various insights and frameworks to offer a roadmap for organizations looking to enhance their data governance practices. By prioritizing governance, investing in infrastructure, and fostering a culture of data stewardship, organizations can harness the power of data to drive innovation and achieve their strategic objectives.
Frequently Asked Questions
What is the role of data stewards in data governance?
Data stewards are responsible for managing data assets, ensuring data quality, and implementing data governance policies. They act as a bridge between data science and policy, ensuring data is used responsibly and ethically.
How does data governance enhance business intelligence?
Effective data governance ensures high data quality, which makes business intelligence more reliable. This leads to better-informed decisions and strategic insights.
What are the main challenges in implementing data governance?
Challenges include understanding the business value of data governance, ownership misconceptions, resource allocation, siloed data, poor data quality, and lack of data control. Addressing these challenges involves clear role definitions, process implementation, and leveraging technology.